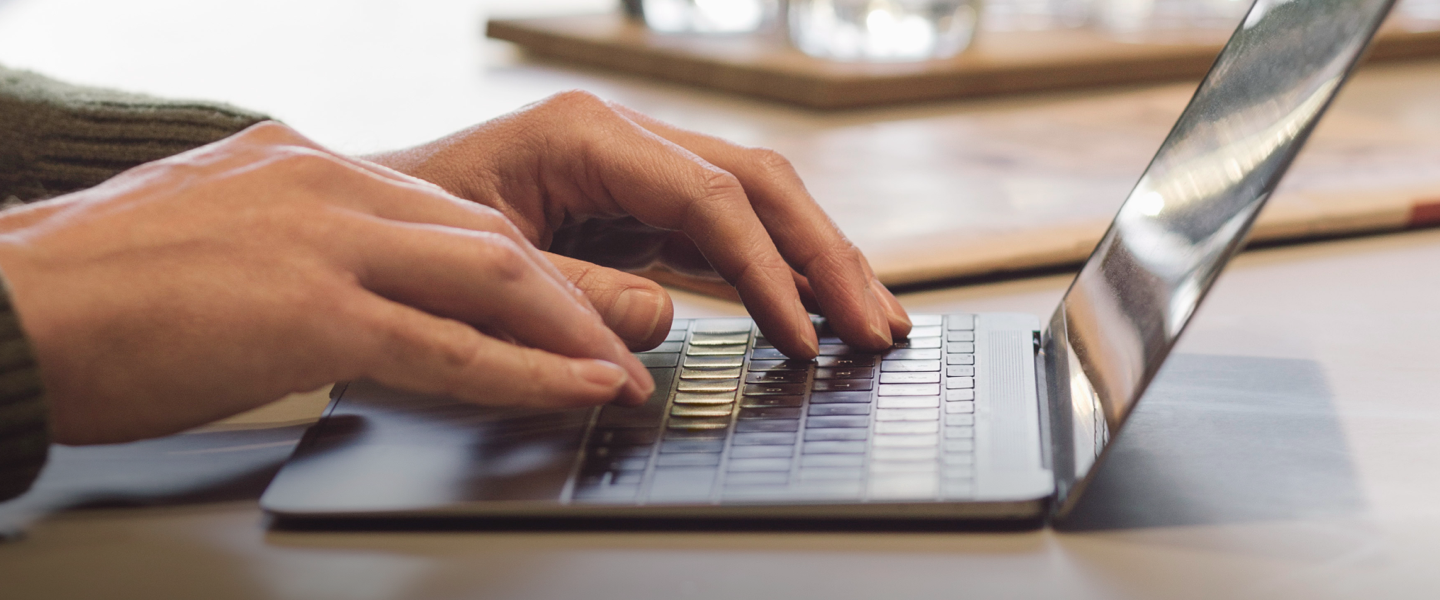
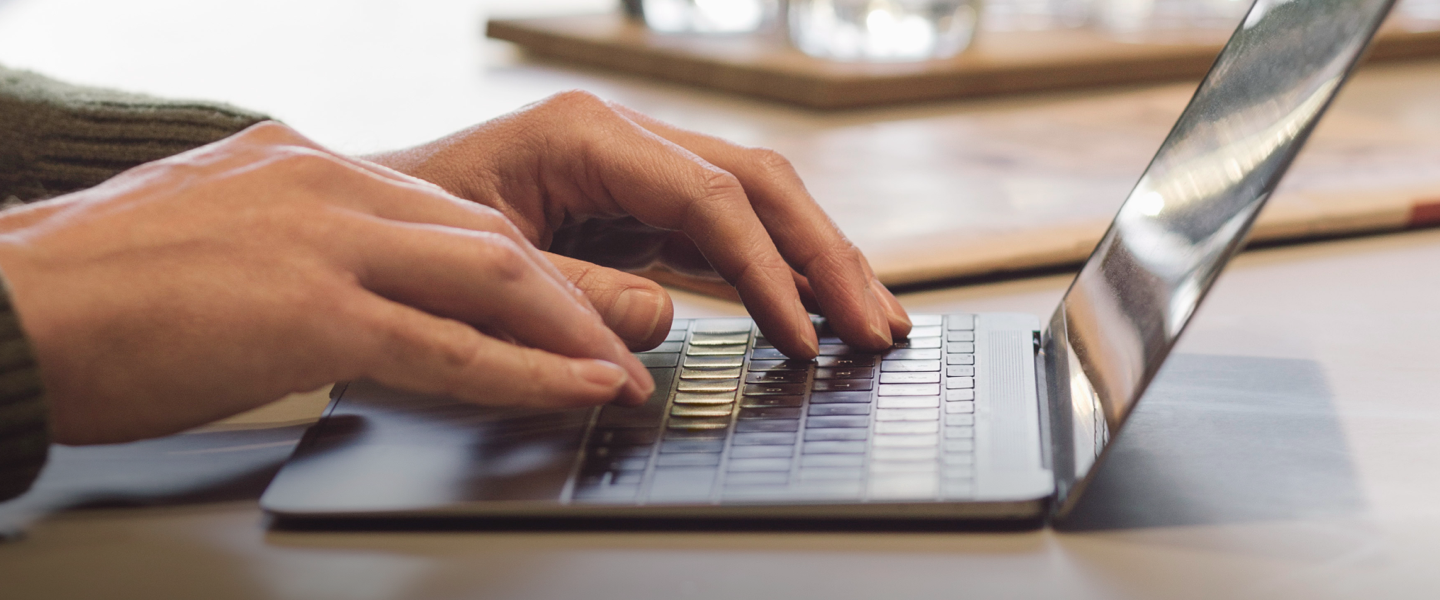
“Two years ago we developed the Zitplaatszoeker (Seat Seeker), to improve passenger distribution in trains. Passengers sometimes felt that a train was overcrowded even though there was still more than enough room at the front or rear. The pandemic has given this crowding and distribution information an extra new function. It's no longer just about indicating where the available seats are; it's about safety and confidence. Information about crowding and distribution helps give passengers the confidence to board a train again and use public transport in these difficult times.
The importance of data is much higher on NS’s agenda now because of COVID-19. Often we already had the data itself but weren't always sure what we could do with it. We have accelerated our transformation into a data-driven organisation. There’s always been a lot of talk about technologies like advanced analytics and artificial intelligence, but now they're being used on an ever bigger scale. We've also started to cooperating far more frequently and effectively, lowering the walls between departments and exchanging data with chain partners.
“Working with the same combined dataset”
In the past, the same data could sometimes be found in different forms at several places within NS. Now we're all working with the same combined dataset. This means we can use information from, for example, weight sensors in the rails and CO2 sensors in the air conditioning system to get an indication of how busy a train is where. It's especially important in times of change to ensure your data management is in good shape, so that you can act quickly.
At the beginning of last year, we didn't expect to see such an acceleration in data digitalization in 2020. Now, one of the most important issues for NS is to regain the public's confidence in travelling by train. The most accurate possible crowd information, based on current data, is very important for this.“Leo van der Meulen - Business Consultant Advanced Analytics NS
”Before COVID-19, NS worked with three-month projections. Now the period we use has become five weeks, and we're very busy developing a model that uses today's data to predict how busy it will be tomorrow. What we'd eventually like to achieve is the ability to improve the crowding prediction on the day itself, for example by using check-in and check-out information from public transport cards or information about mobile phone use in the train.“
”Our department is made up of data scientists who turn large volumes of data into valuable insights and more efficient decision-making. This is done by using models and algorithms, which we embed in processes and products, so that we can further automate and digitalize. The end goal is to make decision-making data-driven and automated wherever possible, supported by a model management tool to operationalize and manage AI (artificial intelligence) models at scale and in a responsible way. We accelerated on this in 2020, so both our internal customers and our suppliers have been able to take advantage of it.
A good example is our fiber roll- out. Previously, creating a design to convert a neighborhood to fiber took at least a week. Using models, we've been able to automate this to a large extent. We base these models on large volumes of data relating to matters such as the exact structure of the streets, the number of trees and the existing cabling. This allows us to quickly calculate how to optimize the installation of new cabling. For example by efficient digging, as little tree drilling as possible, and smart use of existing cabling and equipment in technical buildings.
We are also optimizing our services in the area of customer contact. For example, we are digitizing most customer contacts, such as phone calls, of which we process some 800,000 per month, as well as chats and e-mails. With an AI model, call information is anonymized and categorized, eliminating the need to manually log a call. Relevant information from the conversation is stored automatically as well. On this basis, we are starting a pilot to make the summary available straight away in the customer service system. So when a customer calls again a summary of the previous conversation appears straight away on the customer service agent’s screen. This allows us to better serve our customers and provide more personalized assistance.
“Responsible data use and automated decision-making”
KPN is fully aware of the importance of using of digitalization and artificial intelligence responsibly. We have has set up the KPN Responsible AI Lab together with the national Innovation Center for Artificial Intelligence (ICAI) and the Jheronimus Academy of Data Science (JADS). At this public-private research lab, KPN and JADS work together on PhD research on the cutting edge of data science, technology, decision-making, privacy and compliance, focusing on responsible use of AI. The aim of the KPN Responsible AI Lab is to use AI to develop business solutions that are transparent and handle privacy and personal data with care.“